Training
We qualify you in all aspects of AI. You can choose from an extensive selection of training courses that have been developed to match the services offered in KISSKI. The partners of the KISSKI consortium also open relevant areas of their own training programmes to KISSKI users.
Training courses explicitly designed for KISSKI
In order to register for the courses offered by the GWDG free of charge, you need an AcademicId, which you can easily create.
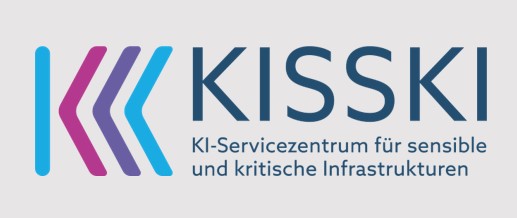
How to KISSKI
Date: 2025-05-06Venue: Göttingen
Organiser: GWDG
You want to learn how to use the KISSKI infrastructure? We will teach how to get onto the front end systems and start batch jobs using SLURM.
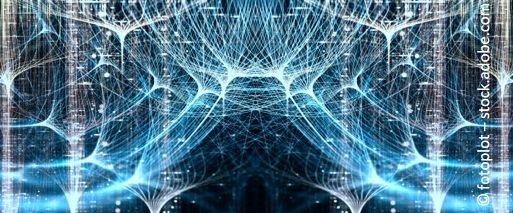
Deep Learning Bootcamp: Building and Deploying AI Models
Date: 2025-06-17 - 2025-06-18Venue: Online
Organiser: GWDG
Introduction to neural networks, model building with TensorFlow and PyTorch and Deployment of AI models.
External courses offered by the KISSKI consortium partners
Thematically appropriate courses from the training courses offered by the institutions participating in the KISSKI consortium. Some of the courses are subject to a fee. In order to register for the GWDG’s course program free of charge, you need an AcademicId, which you can easily create.
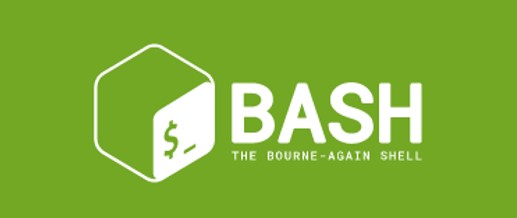
Getting Started with Linux Bash
Date: 2025-06-05Venue: Göttingen
Organiser: GWDG
Are you afraid of the terminal, that black box with the $ signs in front? Become acquainted with the Linux Bash in order to use the power of HPC.
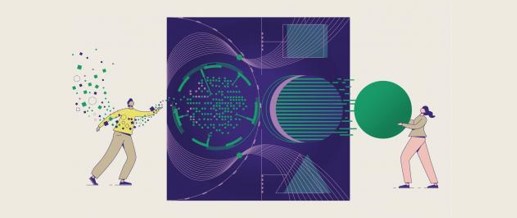
AutoML - Automated Machine Learning
Date: Anytime (self studies)Venue: Online
Organiser: LUH
Learn how to use and design automated approaches for determining Machine Learning (ML) pipelines efficiently
An overview of past courses can be found here.